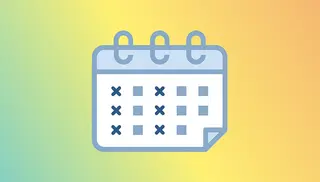
conference
08.08.22
Workshop on Algebraic Geometry, Combinatorics, and Machine Learning
Numerous learning algorithms rely on the geometry and combinatorics of individual hypothesis functions, function classes, their particular parametrization, and the corresponding structure of optimization objectives in parameter and function space, which also depend on the data at hand. In this meeting we will discuss the application of mathematical methods and challenges in Algebraic Geometry and Combinatorics in the realm of Machine Learning and Deep Learning in particular.
Participation is possible both in-person and online.