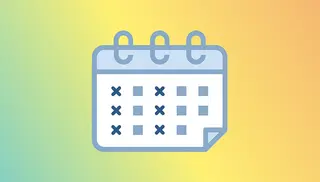
MPI für Mathematik in den Naturwissenschaften Leipzig
Live Stream
conference
31.05.21
02.06.21
Workshop on Software and Applications of Numerical Nonlinear Algebra
The topic of this workshop is solving systems of polynomial equations using numerical algorithms. The participants will learn to work with the software HomotopyContinuation.jl, so that they can use it for their own research problems. In addition, the workshop will feature a series of lectures on the theory of numerical homotopy continuation, as well as contributed talks by Timothy Duff, Alex Heaton, Julia Lindberg and Maggie Regan.