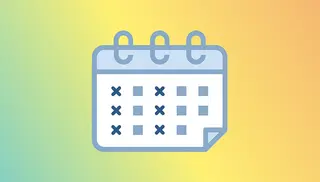
Conference on Mathematics of Machine Learning
The conference will take place in a hybrid format, allowing both in person and online attendance. The submission of contributed talks and posters is now closed.
In recent years, machine learning algorithms have seen an unprecedented success in a variety of fields, ranging from science and engineering to medicine and social sciences. As a result, artificial intelligence has been identified as one of the key technologies for future social and economic advance. With its rising importance also in failure sensitive systems, for example in medical devices and autonomous driving, the need for a systematic understanding of the functionality of machine learning algorithms and for guarantees on their accuracy and precision becomes vital. This calls for the development of a mathematical understanding of the structures and mechanism underlying the success of machine learning techniques. This endeavor has seen a significant increase of interest and efforts in recent years, crucially relying on interdisciplinary interaction, communicating mathematical progress and empirical research. The proposed conference is aimed as a contribution to this rapidly developing field, by bringing together experts from various mathematical areas with shared interest in applications to machine learning and experts from fields such as computer science and biology.