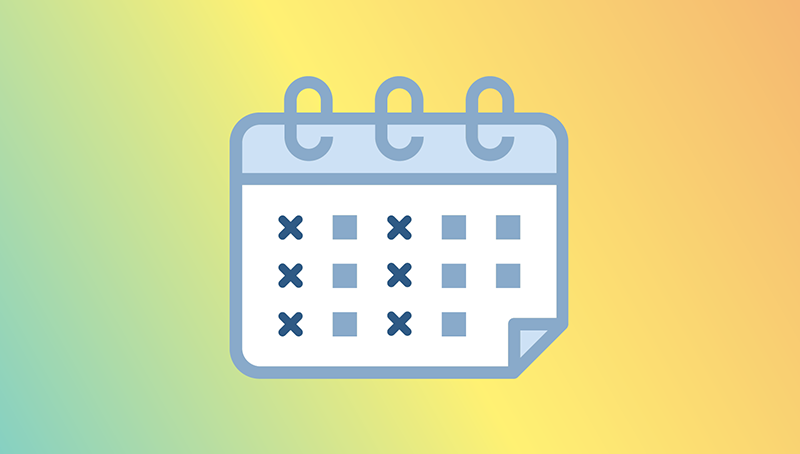
Statistics of Shapes and Geometry of Shape Spaces
Statistics of shapes has a long tradition in medical image analysis and biology. This spans from classical landmark representations and Kendall's shape space to infinite dimensional shape spaces with rich geometric structures. Recent work has added stochastics and statistical inference for stochastic processes to the picture. Shape analysis, shape statistics, and shape stochastics give rise to new research directions in both pure and applied mathematics, and new developments have direct impact in applied fields such as evolutionary biology. The aim of the conference is to establish connections between the communities of shape analysis, differential geometry and statistics for stochastic processes. Focus will be on intersections between geometry including sub-Riemannian geometry, shape analysis, stochastic analysis, and applications in biology, including phylogenetic inference.